Statement on ethics.
Introduction
The rapid development of data science and artificial intelligence (AI) technologies has led to significant advancements across various industries. However, these advancements also raise critical ethical concerns. Addressing these concerns is essential to ensure that the deployment of data and AI technologies is fair, transparent, and beneficial to society.
Ethical Principles in Data and AI
Fairness and Non-Discrimination
Bias and Discrimination: AI systems can perpetuate or even exacerbate biases present in the training data. It is crucial to implement measures that detect and mitigate bias to prevent discrimination based on race, gender, age, or other protected characteristics.
Inclusivity: Ensure that AI technologies serve diverse populations and do not exclude or disadvantage any group.
Transparency and Explainability
Algorithmic Transparency: AI models, especially those used in critical applications like healthcare and criminal justice, should be transparent. Stakeholders need to understand how decisions are made.
Explainability: AI systems should provide clear explanations for their outputs. This is particularly important in high-stakes areas where decisions can significantly impact individuals' lives.
Privacy and Data Protection
Data Privacy: Safeguarding personal data is paramount. AI systems should comply with data protection regulations, such as the GDPR, and employ techniques like data anonymization to protect individuals' privacy.
Informed Consent: Individuals should be informed about how their data will be used and concrete technical measures based on industry standards should be full implemented to ensure thath their explicit non-consent has not been statet before their data is collected and processed.
Accountability
Responsibility: Organizations developing and deploying AI systems must take responsibility for their impacts. There should be clear accountability mechanisms in place to address any adverse outcomes.
Ethical Audits: Regular ethical audits should be conducted to evaluate the fairness, transparency, and privacy aspects of AI systems.
Safety and Security
Robustness: AI systems should be designed to be robust and resilient against attacks and failures. This includes ensuring that they can handle unexpected inputs and scenarios gracefully.
Security: Protect AI systems from malicious attacks that could compromise their integrity or misuse their capabilities.
Human-Centered Design
User-Centric Approach: AI systems should be designed with the end-user in mind, ensuring they enhance human capabilities rather than replace them.
Empowerment: AI should be used to empower individuals, providing tools and insights that enable better decision-making.
Implementing Ethical AI Practices
Ethical Guidelines and Frameworks
Develop and adopt comprehensive ethical guidelines that align with international standards. These guidelines should be integrated into the AI development lifecycle from design to deployment.
Interdisciplinary Collaboration
Encourage collaboration between ethicists, data scientists, engineers, and other stakeholders to address ethical challenges from multiple perspectives.
Continuous Monitoring and Evaluation
Implement mechanisms for the ongoing monitoring and evaluation of AI systems to ensure they adhere to ethical standards over time.
Education and Training
Provide education and training for AI developers and users on ethical issues. This can help foster a culture of ethical awareness and responsibility.
Stakeholder Engagement
Engage with a broad range of stakeholders, including the public, to gather input and feedback on AI systems and their ethical implications.
Conclusion
The ethical deployment of data and AI technologies requires a concerted effort from all stakeholders involved. By adhering to principles of fairness, transparency, privacy, accountability, safety, and human-centered design, we can harness the potential of AI in a way that benefits society while mitigating its risks. Continuous dialogue, education, and proactive measures are essential to navigating the ethical landscape of AI.
Find your data
Datasets at Tofu AI can be searched by the topics the are supposed to represent. By calculating vector embeddings for all data we can match the semantic meaning of every text in a high dimensional vector space with your request.
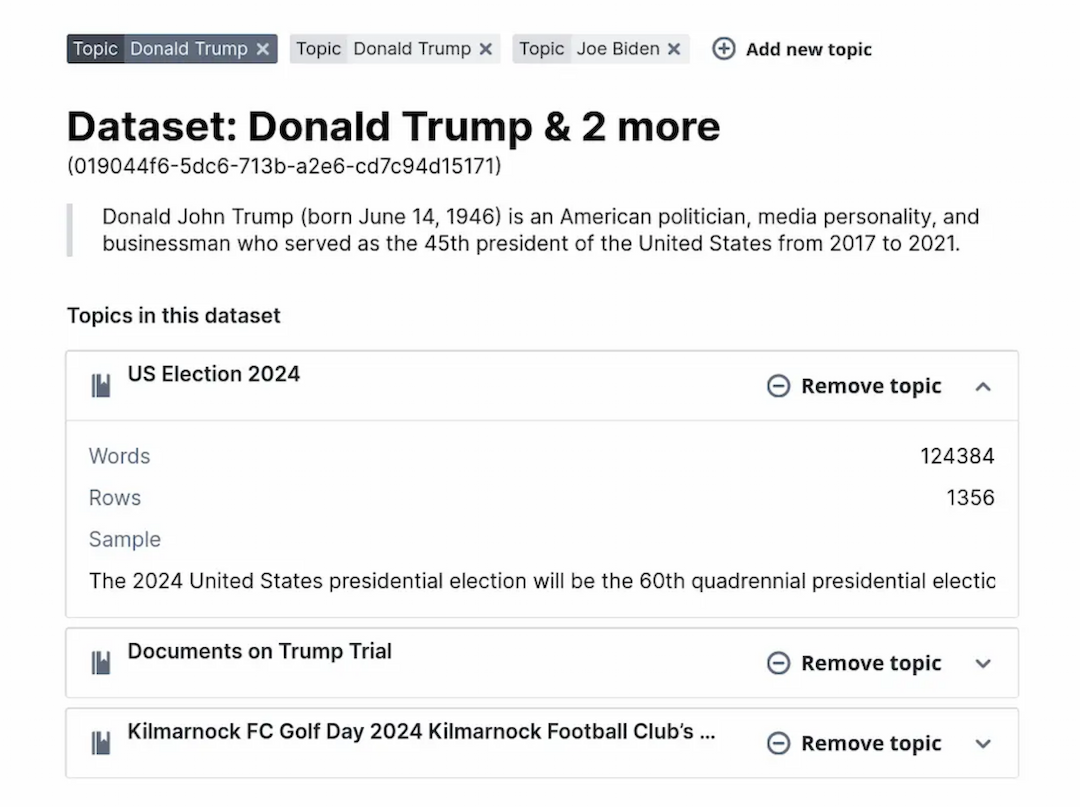